How can colleges use predictive algorithms equitably? A conversation with Dr. Denisa Gándara
Published Sep 13, 2024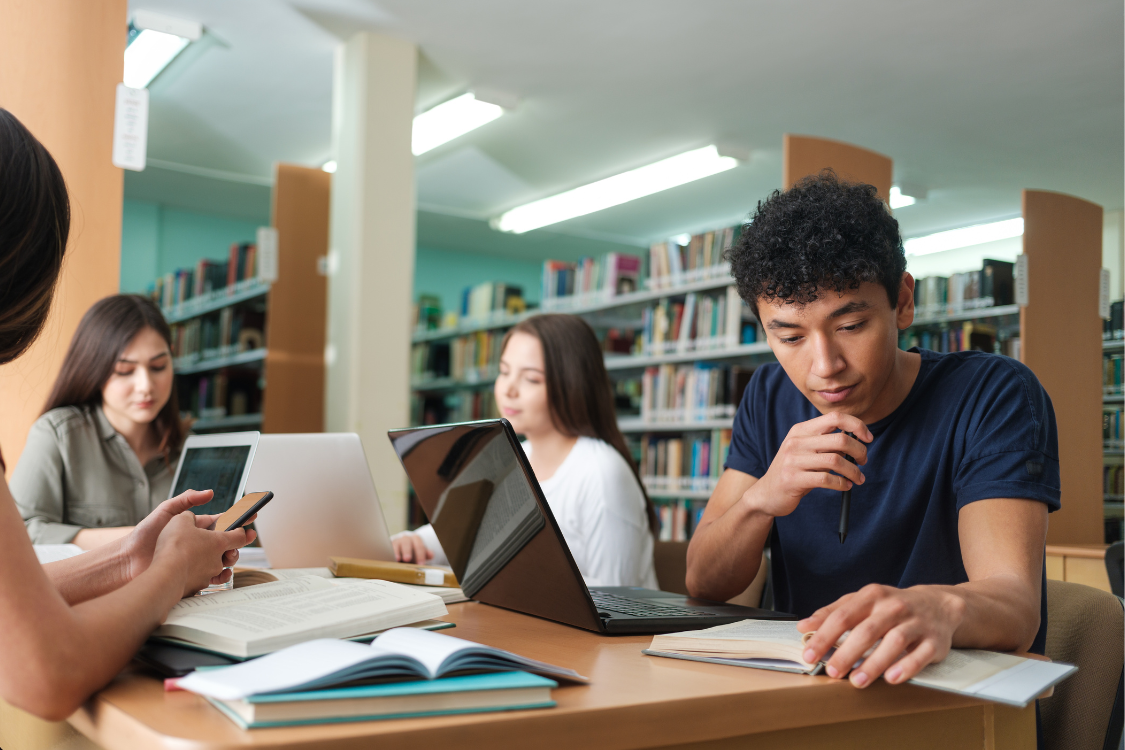
New research reveals that algorithms commonly used by institutions to predict student success may be racially biased against Black and Latinx students. The report, “Inside the Black Box: Detecting and Mitigating Algorithmic Bias Across Racialized Groups in College Student-Success Prediction,” shows that predictive models are more likely to predict failure for Black and Latinx students who succeed, compared to their White and Asian counterparts.
We recently spoke with one of the report’s authors, Dr. Denisa Gándara, an IHEP Board Member and an Associate Professor of Educational Leadership and Policy at The University of Texas at Austin about the implications of her research for institutions seeking to equitably improve student outcomes. This conversation has been edited for length and clarity.
IHEP: What inspired you to focus on algorithmic bias in higher education?
My interest in this topic was sparked by a ProPublica investigation into an algorithm used to predict recidivism in the criminal justice system. The algorithm was more likely to wrongly predict that Black defendants would reoffend compared to White defendants, and these predictions were being used by judges to make sentencing decisions.
Around the same time, institutions were beginning to use predictive models to identify students at risk of not graduating and I wondered if these tools might be biased as well. This could be racial bias, which is the focus of this research, but also bias based on students’ gender, field of study, location, or socioeconomic status.
When I got the opportunity to partner with Hadis Anahideh, an industrial engineer at the University of Illinois who specializes in fair prediction algorithms, we set out to evaluate these tools in higher education. These models are increasingly being used to inform decisions about student recruitment, admissions, and supports.
IHEP: What are the most promising strategies for institutions to use these predictive models equitably?
Transparency, accountability, and racial consciousness are crucial to ensuring the equitable usage of predictive models. People often view these algorithms as objective. But these models are based on historical data, so the outputs reflect patterns in these data, including societal inequities.
When a model predicts that Black or Latinx students are more likely to fail, how users interpret that information depends on their racial consciousness and data literacy. Faculty and staff who are aware of their own potential biases and the societal biases embedded in the data are better equipped to use these models to support rather than hinder equity in student outcomes. For example, if faculty hold deficit assumptions about these students’ ability to succeed, they may dismiss those students as a lost cause. On the other hand, they could consider their role in proving the model wrong.
Institutions also should avoid educational tracking, the practice of diverting students into courses or majors where they have a higher likelihood of success to improve graduation rates. This may exacerbate inequities beyond higher education, including economic outcomes.
Whenever possible, institutional researchers or external experts should audit these predictive models for bias. Institutions who develop their own models in-house, primarily well-resourced, four-year research universities, should rigorously evaluate them to identify areas for improvement on their campuses. Some institutions rely on vendors for predictive models that are proprietary, which can pose challenges. Those institutions should advocate for greater transparency from vendors in order to conduct external evaluations to ensure fairness and mitigate bias.
IHEP: How can institutions build data literacy among faculty and staff to ensure that predictive models are used responsibly?
One strategy is to integrate data literacy training into existing professional development initiatives to help faculty and staff better understand the connections between the data and their day-to-day work with students.
Another important step is giving clear guidance within the user interfaces of these tools on how to interpret the outputs, identify potential biases, and think critically about the “why” behind the data to inform policy and practices changes. There are good examples of this already including from EAB.
IHEP: If campuses can effectively mitigate bias in these models, what are the opportunities for these models to promote equitable outcomes in higher education?
Predictive models can be powerful tools to help institutions identify the students who need support the most. For example, they can prompt academic advisors to reach out to students at risk of not passing a class or graduating to offer additional support like tutoring or financial assistance.
Paired with robust support services and a commitment to racial equity and critical data use, colleges can fully realize the potential of predictive analytics to promote equitable postsecondary outcomes.